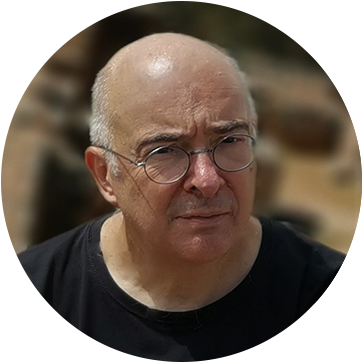
Eric Moulines
Ecole polytechnique, France
21st July 2022, 4:00pm - 5:00pm (GST)
Title: | Efficient federated Bayesian sampling by Stochastic Averaging Langevin Dynamics |
Abstract: | In this work, we develop new methods for Bayesian computation in a federated learning context (FL). While there are a variety of distributed MCMC algorithms, few have been developed to address the specific constraints of FL, such as data privacy, communication bottleneck, and statistical heterogeneity. To tackle these issues, we propose SALaD, a MCMC algorithm that combines the ideas of Stochastic Langevin Gradient Dynamics and Federated Averaging. In each round, each client executes SGLD to update its local parameter, which is sent to a central server. The central server then in turn sends the average of the local parameters to the clients. However, this method may suffer from the high variance of the stochastic gradients used by local SGLD and the heterogeneity of the data, which hinders and/or slows down convergence. To address these issues, we propose three alternatives based on a combination of control variates and bias reduction techniques for which theoretical improvements are derived. We illustrate our findings using several FL benchmarks for Bayesian inference. |
Bio: | Eric Moulines earned a degree in engineering (1984) from the Ecole Polytechnique, Paris, and a doctorate in electrical engineering (1990) from the Ecole Nationale Supérieure des Télécommunication. In 1990, he joined the Signal and Image Processing Department at Télécom ParisTech, where he was appointed full professor in 1996. In 2015, he joined the Centre for Applied Mathematics at Ecole Polytechnique, where he is currently Professor of Statistics. |