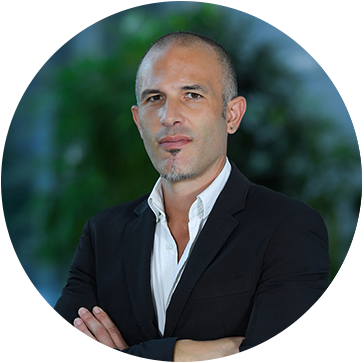
Emilio Porcu
Professor of Statistics at Khalifa University
17th November 2022, 4:00pm - 5:00pm GST
Title: | Kernel Methods in Probabilistic Learning: Stein Discrepancies on Manifolds) |
Abstract: | Reproducing Kernel Hilbert Space methods have become ubiquitous in both statistical learning and machine learning communities. My talk illustrates Stein methods within the planet of Quantisation, being the act of approximating a given probability distribution (think of a picture on the unit square) with a linear combination of Dirac delta functions (a coarse version of the picture). We provide a comparison with quasi Monte Carlo and with MCMC methods in terms of statistical accuracy. The latter being measured in terms of upper bounds for the average worst case error. We also inspect computational scalability of the proposed methods. |
Bio: | Emilio Porcu got his PhD in statistics in 2005. He became a Full professor in 2012, and Chair Professor at Newcastle University and then Trinity College. He is Professor of Statistics at Khalifa University since August 2020. |