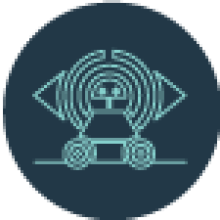
Perception and Computer Vision
We are focused on developing cutting-edge solutions that enable our robots to understand their surroundings by leveraging classical and modern computer vision methods.
Our research domains include:
- Object detection and classification techniques in challenging light conditions
- Object identification and tracking from different perspectives and distances
- Semantic segmentation for areas like autonomous driving, scene understanding and robot manipulation
- Localization in both global and local positioning systems
- Geometric and semantic mapping for aerial and ground vehicles
- Machine learning techniques for underwater computer vision with limited datasets
- Visual-based navigation systems for UAVs
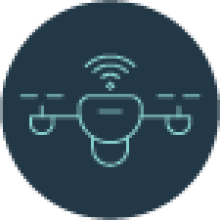
Communication and Networking
We study and develop enabling communications and networking technologies, algorithms, and protocols for cooperation, coordination, and distributed perception, control and decision-making in a group of aerial, terrestrial, marine, and underwater robots.
Our research domains include:
- Wireless communication and PHY layer technologies for networked robotics
- Infrastructure-to-robot and robot-to-robot communication
- 5G/6G-enabled networked robotics
- Communications and networking for swarm coordination and cooperation
- Data link, Network, and Transport protocols for groups of robots
- AI-driven communications for networked robotics
- Quality of service, security, and communications robustness in networked robotics
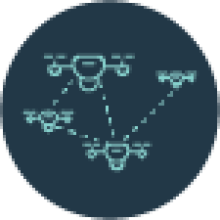
Swarm and Coordination
We draw inspiration from classical multi-robot systems and biology to develop breakthrough algorithms for cooperative, robust and distributed decision making and task allocation, group navigation, area coverage and scanning, and human swarm interaction for all robotics domains.
Our research domains include:
- Hybrid swarms across aerial, terrestrial, marine, underwater robots
- Cooperative motion and group navigation: flocking and formation
- Task allocation by means of decision trees and auction based approaches
- Distributed online area coverage and scanning
- Human swarm interaction
- Software applications and utilities for swarm generalization
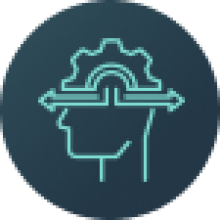
Control and Decision Making
Beginning with existing research in decision-making, trajectory planning, motion planning, control, state estimation, and machine learning, our work employs state-of-the-art technologies in new and challenging domains.
Our research domains include:
- Stabilization, control, and navigation of unmanned aerial vehicles (UAVs), terrestrial and marine vehicles in structured and unstructured domains
- Trajectory and motion planning with constraints originating from either the robot platform used or the environment. On-board version for unknown environments.
- Hybridization of decentralized control with swarm robotics principles. Distributed algorithms for synchronization and for task allocation in multi-robot systems
- Machine learning and reinforcement learning for decision-making in unseen environments
- Autonomous driving in unstructured environments, such as the desert
- State estimation methodologies for robot state estimation and for external target tracking
- Control methodologies for high speed and/or agile maneuvering