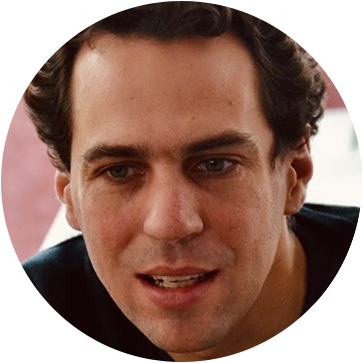
Panayotis Mertikopoulos
French National Center for Scientific Research, Laboratoire d'Informatique de Grenoble
13th April 2023, 2:00pm - 3:00pm (GST)
Title: | Training models with a min-max loss landscape |
Abstract: | This talk aims to survey the triple-point interface between optimization, game theory, and dynamical systems. We will begin by discussing how the ordinary differential equation (ODE) method of stochastic approximation can be used to analyze the trajectories of a wide array of stochastic first-order algorithms in non-convex minimization problems and games (including extra-gradient and optimistic gradient methods). This methodology allows us to derive a range of conditions guaranteeing convergence to min-max stable points while avoiding unstable points and periodic orbits. However, despite these encouraging results, the overall situation can be considerably more involved, and the sequence of play may converge with arbitrarily high probability to lower-dimensional manifolds that are in no way unilaterally stable (or even stationary). "Spurious attractors" of this type can arise even in simple two-player zero-sum games with one-dimensional action sets and polynomial losses of degree 4, a fact which highlights the fundamental gap between minimization and saddle-point problems. |
Bio: | Panayotis Mertikopoulos is a principal researcher at the French National Center for Scientific Research (CNRS). After graduating valedictorian from the University of Athens in 2003, he received his MSc and MPhil degrees in mathematics in 2005 from Brown University, and his PhD degree from the University of Athens in 2010. He then spent one year as a post-doctoral fellow at École Polytechnique in Paris before joining the CNRS in 2011. Since 2011, he has held visiting or part-time positions at UC Berkeley, EPFL and, more recently, Criteo AI Lab. |