After a long AI winter of more than two decades, Machine Learning (ML) is witnessing a renewed surge of interest across a wide range of sectors. The arrival of new and powerful computational resources is gradually expanding the horizons for Machine Learning. ML is now establishing benchmarks of excellence with regard to data modelling. Thanks to its ability to learn and act without explicit programming and with minimal human intervention, ML has already enhanced efficiencies in domains such as image classification, game playing, autonomous driving, medical diagnosis, and even financial system management. These achievements are only the tip of the iceberg
At Technology Innovation Institute’s (TII’s) Directed Energy Research Center (DERC), we believe in the significance of early adoption of ML. We are engaged in integrating its techniques to foster smooth and rapid implementation of research plans, specifically those related to Signal Processing (SP). In addition to DERC, such integration can also significantly benefit several other teams across TII. Through integrating Machine Learning into our operations, we are focused on further catalysing our research activities in this new decade. TII is also expanding several existing research projects and rolling out initiatives to define the best approaches and strategies to incorporate and apply ML to several areas of its research agenda.
For instance, after the COVID-19 outbreak in Q2 2020, and as a part of the Citizen Science UAE initiative [1] that aims to highlight the need for more specialised equipment, data-driven insights, and relevant technologies, Convolutional Neural Networks (CNN) were developed and trained to predict COVID-19 induced pneumonia from chest x-ray scans using Deep Learning [2]. With a supra-human accuracy of 97.5%, such systems prove the promising potential of ML in image processing tasks and opens up new possibilities for multi-disciplinary scientific collaboration.
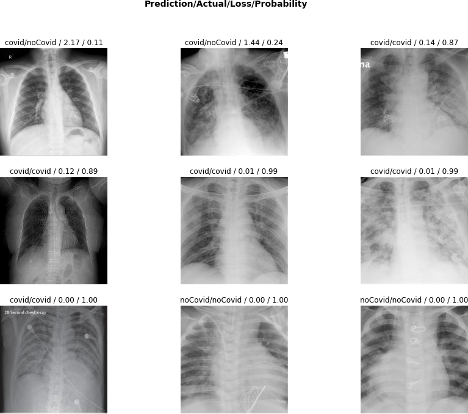
Figure 1. Predictions performed by CNN over chest x-rays. The diagnosis probabilities may be seen on top of each image.
In addition, significant advances in electromagnetic security have been achieved recently, as researchers from DERC reassessed the threat posed by electromagnetic information leakage from critical infrastructure systems [3,4]. DERC showed how cutting-edge ML-based approaches for Signal and Image Processing allow for the successful retrieval of up to 68% of the text information from compromising electromagnetic emanations of video interfaces - such a feat was considered impossible just a few years ago. This contribution opens up possibilities for automatic emissivity evaluations of electronic devices to preserve confidentiality and prevent information leakage.
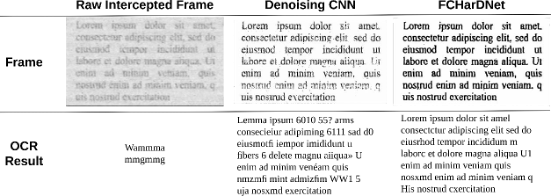
Figure 2. Original acquired and processed video frames compared to images processed with two different CNNs and text information extraction using Optical Character Recognition (OCR). The processed data originates from leaked electromagnetic emanations of a video interface.
Furthermore, research on the processing of RF communication signals [5] has shown the potential ML holds in detecting and classifying UAVs using RF fingerprints. This is an important application due to the intrusion threat posed to critical infrastructures by the widespread availability of low-cost UAVs. Detection using CNN can reach accuracies of up to 95%. Such results enable the deployment of real-time RF spectrum monitoring stations capable of early detection of foreign UAVs to safeguard critical infrastructures. Moreover, such station provide great support for cognitive radios that can reuse the spectrum dynamically for navigation and location purposes to overcome spectrum congestion.
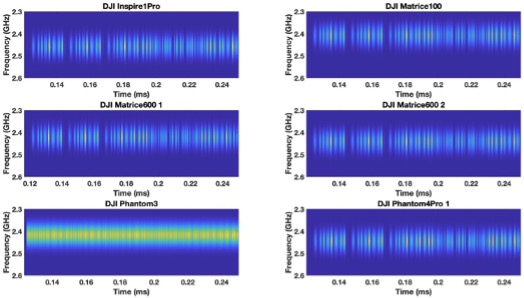
Figure 3. Spectrograms of the RF fingerprint of different UAV models as classified by a CNN.
Ultimately, ML presents a large untapped potential to find solutions to some of the most relevant scientific challenges of our time. Several computationally intensive and decision-based tasks can be efficiently deployed and managed by training Neural Networks to pick up and detect hidden patterns that might otherwise be imperceptible to human beings. Integrating ML into Signal Processing is a guaranteed gateway to creating a transformational impact across several research disciplines within a relatively short time frame for DERC.
References
- Citizen Science. http://www.citizenscience.ae/. Accessed 10 January 2021
- CitizenScienceUAE. “CNN for the Classification of Chest X-ray Scans.” Citizen Science Github, https://github.com/CitizenScienceUAE/covid19_xray_pytoch. Accessed 10 January 2021.
- Deep Learning-based Denoising of TEMPEST Images for Efficient Optical Character Recognition. TechRxiv. Preprint. https://doi.org/10.36227/techrxiv.12488672.v1
- Re-Assessment of the TEMPEST Threat in the Era of Machine Learning and OCR. Submitted to IEEE International Symposium on EMC, 2021, https://www.emcs.org/ieee-symposia-schedule.html
- UAVs based on Spectrum Monitoring and Deep Learning in Negative SNR Conditions, XXXIV General Assembly and Scientific Symposium (GASS) of the International Union of Radio Science, 2021.