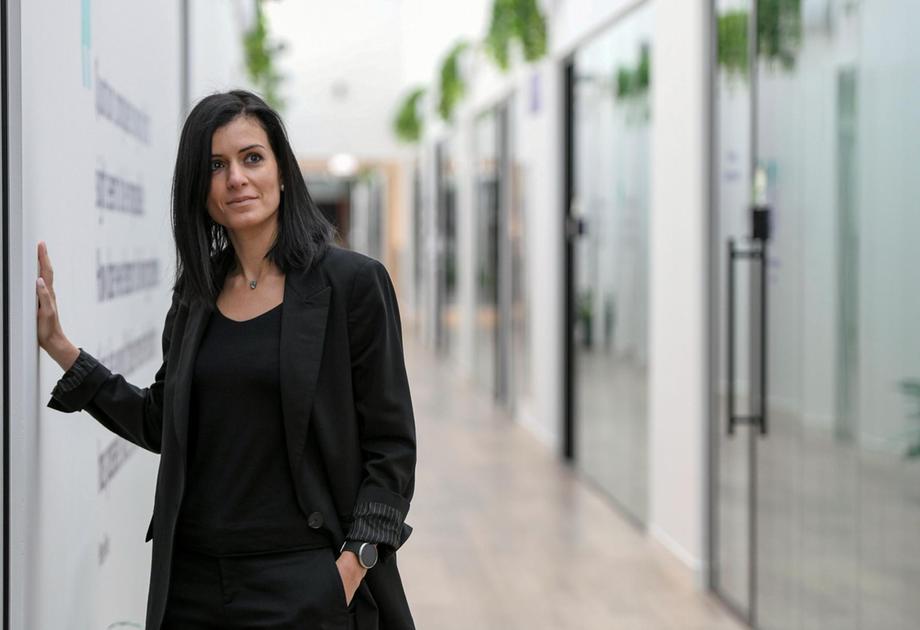
By Dr. Najwa Aaraj, Chief Researcher, Cryptography Research Center and Acting Chief Researcher, Autonomous Robotics Research Center
The human brain has been an object of considerable fascination since the beginning of time. Its unique ability to multitask with agility while still operating on far less energy – roughly 20 watts of power on average, which is about half the energy required by a standard laptop and 0.00007% of the supercomputer Fugaku's power supply – is often referred to with incredulity. That is not the end of it, either. While supercomputers need elaborate cooling systems, the brain keeps functioning efficiently at 37°C. With information and communication technologies currently accounting for roughly 20% to 35% of global energy consumption and given the surge in the number of inter-connected devices, there is now a growing interest in neuromorphic technologies or computing.
Neuromorphic computing refers to the use of very-large-scale integration systems containing electronic analog circuits to mimic neuro-biological architectures present in the nervous system How it works is by mimicking the physics of the human brain through establishing what are known as Spiking Neural Networks, where spikes from individual electronic neurons activate other neurons down a cascading chain. This is similar to the manner in which the brain sends and receives signals from biological neurons that spark or recognise movement and sensations in our bodies. Neuromorphic computing, specifically, Spiking Neural Networks have become very popular as an energy-efficient alternative for implementing standard artificial intelligence tasks. Spikes or binary events drive communication and computation in Spiking Neural Networks which offer the benefit of event-driven hardware operation. This makes them attractive for real-time applications where power consumption and memory bandwidth are important factors.
While the human brain collects information from within the body and sends out impulses to the neurons, traditional computers deal with the challenge of bottle necks because of the separation between the CPU and the memory (RAM). This is no longer the case with neuromorphic computing – where the computation is done in analog – meaning, through memory. In addition to eliminating latency and power drain, neuromorphic computing opens up possibilities to carry out complex computation.
The Spiking Neural Network (SNN) model emulates natural neural networks present in biological brains and simulates the natural learning processes by dynamically mapping the synapses between artificial neurons in response to stimuli. SNNs can convey information in both the temporal and spatial way just as the brain can whereas conventional computing is based on transistors that are either on or off.
The core advantage we seek to achieve with neuromorphic computing is to get computers to think creatively and nimbly, start recognising people or objects they have not seen earlier, and recalibrate their actions as appropriate. Clearly, we are still some distance from witnessing such high-performing supercomputers. Although AI can today tackle several challenges better than human beings, it is still unable to analyse cause and effect and quickly pivot to accommodate changing configurations or new elements of a problem.
However, the research is promising. Last year, for instance, Intel showed off a neuromorphic robot that can see and recognise unknown objects based on just one example, unlike traditional models that require extensive instruction and data. Similarly, Intel and Cornell University debuted mathematical algorithms, used on Intel's neuromorphic text chip, Loihi, that closely replicate how the brain "smells" something. Loihi was able to distinguish up to 10 different smells. This AI capability could see applications in airport security, smoke and carbon monoxide detection, and boost quality control in factories. Accenture Labs later demonstrated "Automotive Voice Command," leveraging a Loihi-based experiment to bring voice, gesture, and contextual intelligence command capabilities to vehicles without draining batteries.
Although Neuromorphic computing has been around since the 1980s, in recent years, it has gained traction due to power-hungry drones, a surge in autonomous robotics innovations, and the growing uptake for AI and machine learning. Unfortunately, the corresponding hardware advancements to launch such AI are based on the von Neumann architecture that is known to drain energy and time through communicating information between the memory and processors.
Gartner predicts traditional computing technologies built on legacy semiconductor architecture will hit a digital wall by 2025 and force a shift to new paradigms, including neuromorphic computing. Emergen Research, a syndicated research and consulting firm focusing on the upcoming emerging industries, says the global neuromorphic processing market will reach US$11.29 billion by 2027.
Today’s legacy chipsets may not efficiently enable the deployment of AI to IoT devices and thereby render on-device intelligence and inference difficult when it comes to robustness to adversarial attacks, explainability, and energy efficiency – all of which are critical in enabling the viability of autonomous vehicles, to name just one example.
Currently, von Neumann architecture based standard computers running machine intelligence tasks need more than 10 times the power of the human brain although they do not solve problems anywhere near the same degree of complexity as the human brain can. Or indeed, with as much comprehension. Explainability also suffers in such scenarios.
To complete computation and data intensive tasks, edge devices like smartphones currently have to transfer the data to a centralised cloud-based system, which processes the data and feeds the result back to the device – subjecting data to security and privacy risks. This architecture could defeat the very raison d'être of neuromorphic systems that aim to rule out this data transfer and enable the processing to take place within the device itself. As we race ahead with more advanced algorithms and emerging neuromorphic competencies, legacy architecture will need to give way to state-of-the-art neuromorphic architecture.
Neuromorphic systems are likely to develop better AIs as they're more comfortable with other types of complex problems. Causality and non-linear thinking are relatively nascent in neuromorphic computing systems that will now need to come up to speed to deploy the solutions that already exist and are surprisingly mature. Inevitably, these will only improve in the future and vastly expand AI applications.
Neuromorphic technology may well require a paradigm shift in the development of hardware and software. We need to work with neuromorphic devices that comply with a new generation of memory, storage and sensor technology. With an eye on future-proofing such technology and assessing the performance of new architectures to further enhance their capabilities, it is essential to create an Application Programming Interface (API), as well as programming models and languages.
Which might just help us to finally plumb the depths of AI as we know it and answer that million-dollar question - about whether through emulating the human brain in silicon, scientists will be any closer to creating social consciousness and ethics in machines.