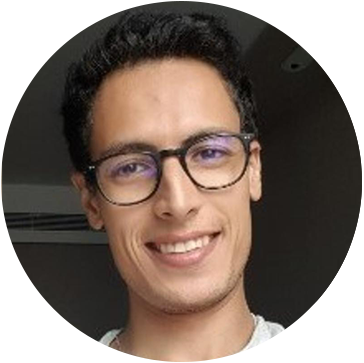
Soufiane Hayou
Assistant Professor of mathematics at the National University of Singapore
15th Feb 2023, 11:00am - 12:00pm (GST)
Title: | Principled scaling of deep neural networks |
Abstract: | Neural networks have achieved impressive performance in many applications such as image recognition and generation, and speech recognition. State-of-the-art performance is usually achieved via a series of engineered modifications to existing neural architectures and their training procedures. However, a common feature of these systems is their large-scale nature. Indeed, modern neural networks usually contain millions - if not billions - of trainable parameters, and empirical evaluations (generally) support the claim that increasing the scale of neural networks (e.g. width and depth in a multi-layer perceptron) enhances the model performance. However, given a neural network model, it is not straightforward to address the crucial question `how do we scale the network?'. In this talk, I will discuss certain properties of large-scale neural networks and show how we can leverage different mathematical results to build robust networks with empirically confirmed benefits. |
Bio: | Soufiane Hayou obtained his PhD in statistics in 2021 from Oxford where he was advised by Arnaud Doucet and Judith Rousseau. He graduated from Ecole Polytechnique in Paris before joining Oxford. During his PhD, he worked mainly on the theory of randomly initialized infinite-width neural networks on topics including the impact of the hyperparameters (variance of the weights, activation function) and the architecture (fully-connected, convolutional, skip connections) on how the 'geometric' information propagates inside the network. He is currently a Peng Tsu Ann Assistant Professor of mathematics at the National University of Singapore. |