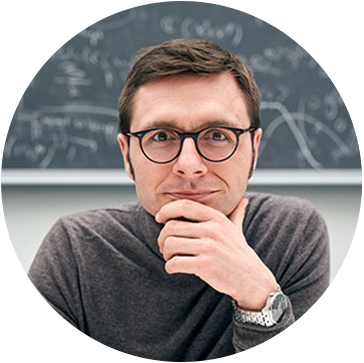
Ivan Dokmanić
University of Basel and University of Illinois at Urbana-Champaign
23rd August 2022, 4:00pm - 5:00pm (GST)
Title: | Universal Geometry and Statistical Mechanics of Learning on Graphs |
Abstract: | The recent success of hyperbolic geometry in learning from hierarchical data is a striking illustration of the power of non-Euclidean feature spaces. Nonetheless, many real-world datasets cannot be represented in hyperbolic nor the various mixed-curvature spaces without significant distortion. The looming question is: is there a simple “universal" feature space that faithfully represents datasets sampled from vastly different metric spaces $X$? We show that, curiously, no Riemannian manifold does the job, but Delon and Desolneux’s space of univariate Gaussian mixtures with a transport metric does. Further, the corresponding representations can be computed by small _probabilistic transformers_ which provably embed any finite metric graph sampled from $X$. This sweeping generalization of a number of recent works on graph embeddings opens the door to “universal” ML pipelines. I will then interpret metric graphs as defining notions of regularity for data supported on them. The best technology to exploit these notions of regularity are graph neural networks, but little is understood about the way the interaction between the two pieces of the puzzle—the graph and the data—impacts generalization in GNNs. I’ll show how tools from statistical physics let us precisely characterize learning curves in synthetic graph–data models and reveal various emergent phenomena such as the marked difference in generalization between homophilic and heterophilic graphs, and, for the first time, the double descent in graph neural networks. These insights pleasingly translate into recipes to improve performance of state-of-the-art networks on large graphs. |
Bio: | Ivan Dokmanić is an Associate Professor in the Department of Mathematics and Computer Science at the University of Basel, Switzerland. From 2016 to 2019 he was an Assistant Professor in the Coordinated Science Laboratory at the University of Illinois at Urbana-Champaign, where he now holds an adjunct appointment. He received a diploma in electrical engineering from the University of Zagreb in 2007, a PhD in computer science from EPFL in 2015, and did a postdoc at Institut Langevin and Ecole Normale Supérieure in Paris between 2015 and 2016. Before that he was a teaching assistant at the University of Zagreb, a codec developer for MainConcept AG, Aachen, and a digital audio effects designer for Little Endian Ltd., Zagreb. His research interests lie between inverse problems, machine learning, and signal processing. He received the Best Student Paper Award at ICASSP 2011, a Google PhD Fellowship, an EPFL Outstanding Doctoral Thesis Award and a Google Faculty Research Award. In 2019 the European Research Council (ERC) awarded him a Starting Grant. |